Does Bayes’ Approach Mimic The Natural Learning Process?
We have seen Bayes’ theorem before. It is a way of updating the chance of an event to occur based on what is known as a prior probability. Check the formula, in case you forgot it because we will use it again.
We do an exercise to illustrate today’s storyline, courtesy – a course in Bayesian statistics run by the University of Canterbury. You learn about a café in town. The shop has two baristas, one experienced and one less experienced. You also came to know that the experienced makes excellent coffee 80% of the time, good coffee 15% and average coffee 5% of the time. For the less experienced, the performance stats are 20%, 50% and 30%, respectively. Your task is to go to the cafe, order, enjoy the coffee and identify the barista.
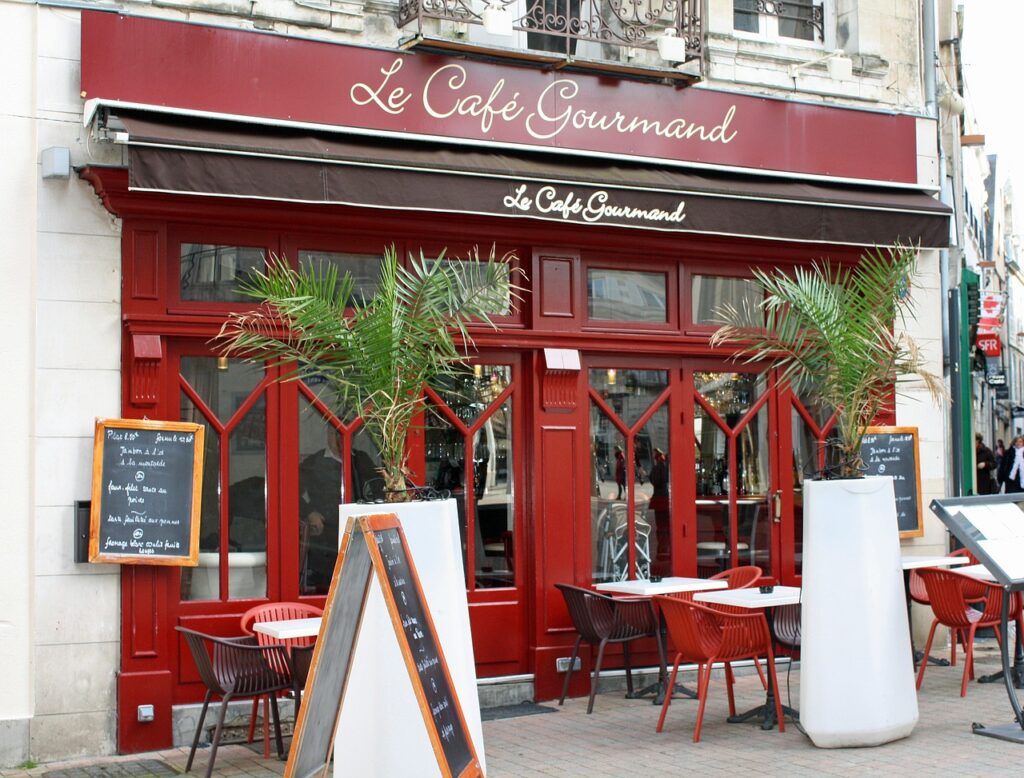
You order a cup of coffee and find it an excellent one. Is this prepared by A? Let’s use Bayes’ equation. But, where do you start? You need a prior probability to begin. One way is to assign a 50-50 chance of encountering A on that day. Another way is to use your knowledge about the workdays per week, which sounds better than 50-50.
The chance that A made your coffee, given that it was an excellent coffee:
P(A|E) = 0.8 x (5/7) / [0.8 x (5/7) + 0.2 x (2/7)] = 0.90
There is a 90% chance that A prepared your coffee. In other words, the initial estimate of (5/7), based on the work pattern, is updated to a higher value.
You got time to spend on another cup of coffee, and it turned out to be an average one! Now you update your estimate using the following calculation,
= 0.05 x (0.9) / [0.05 x (0.9) + 0.3 x (0.1)] = 0.625
Note that the new prior probability is 0.9 and not 5/7. The updated chance (also known as the posterior probability) is still in favour of A but reduced from 90% to 63%.
The true scientist in you tries another coffee, and the outcome was an excellent coffee, and the updated chance is now 87%. At this stage, you decided to end the exercise and conclude that the barista of that day was the experienced one.
Natural Learning Process
This process of updating knowledge based on new information is considered by many as natural learning. I would view this as the ideal learning process, which rarely happens, naturally. In real life, most of us like to carry on the baggage of knowledge – be it from our parents, peers or other dogmatic texts, and resists every opportunity to update. In other words, you ignore new information or the information that does not tally with the existing.
In my opinion, scientific training is the only way to counter the tendency to carry on the baggage. The evidence-based worldview is not natural and needs forceful cultivation.
Back to Café
In the barista story, you may be wondering why the process repeated a few times, and the enquirer settled for a lower probability than what occurred after the first cup. To get the answer, consider the exact three cups of coffee but in a different order, say the average one first. What difference do you see?
Does Bayes’ Approach Mimic The Natural Learning Process? Read More »