Road Safety in India – Survival rate
In the final episode of accident data analysis, we will go into the remaining key stats – injuries and fatalities – and postulate a potential problem with the interpretation, i.e. data registration. But first, a plot of the number of injuries per population.
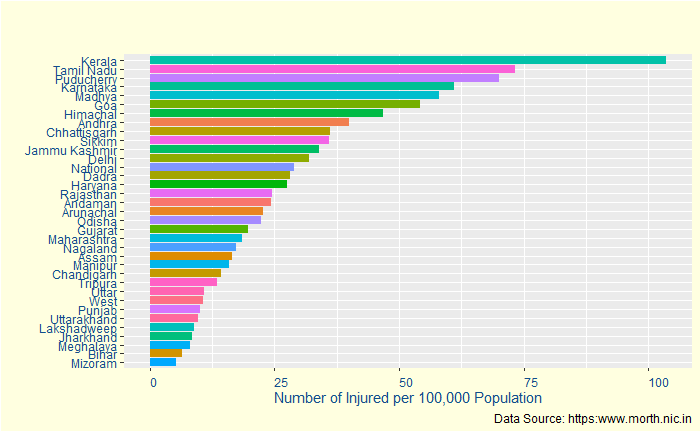
Kerala is now 33% more than the nearest rival, almost suggesting it is the most dangerous state for a passenger. But is that entirely true? Let’s see the following statistic – the fatalities per 100,000 population.
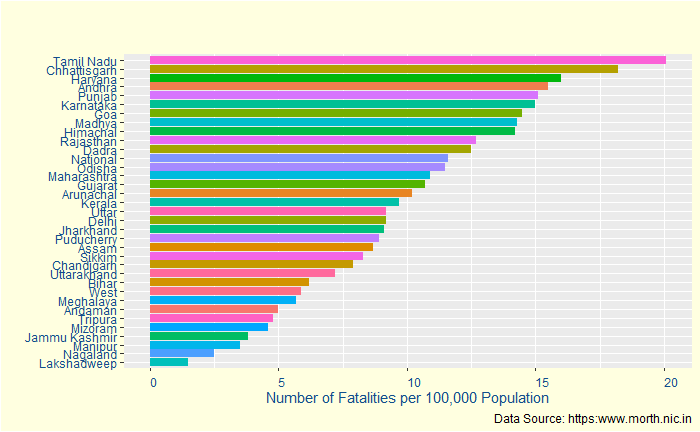
Strangely, it moves down to the 16th. Puducherry, which is third in injuries, also goes down. To understand this better, let’s define survival rate = the number of injured / (number of injured + number of dead).
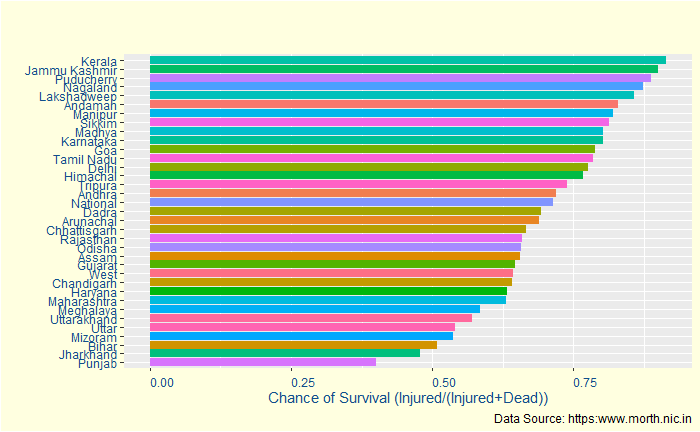
Yes, Kerala has a > 90% survival chance after an accident. It may indicate a few things:
1) Kerala has better accident care for the injured (that prevents them from dying)
2) Kerala has more proportion of low-intensity accidents compared to other states
3) Kerala’s registration system is more thorough in recording incidents. And higher survival rate is an artefact of having a higher reporting rate of all incidents, however minor it could be.
Not so fast
When you are about to conclude data collection, here is another one: the proportion of grievously injured people among the total Injured.
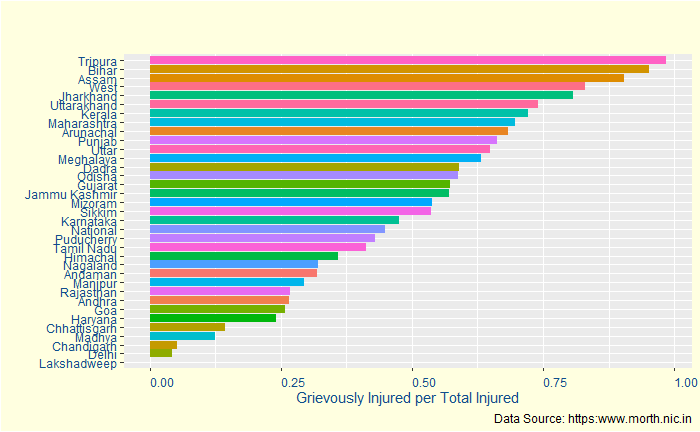
Almost 75% of the injured are seriously injured. So to conclude, Kerala remains one the most dangerous for road safety, but most of the injured are somehow saved, despite the severity.
Road Safety in India – Survival rate Read More »