Here are Kaplan – Meier plots for males and females taken from the results of a cancer study. The data comes from the ‘BrainCancer’ dataset from the R library ISLR2. It contains the survival times for patients with primary brain tumours undergoing treatment.

At first glance, it appears that females were doing better, up to about 50 months, until the two lines merged. The question is: is the difference (between the two survival plots) statistically significant?
You may think of using two-sample t-tests comparing the means of survival times. But the presence of censoring makes life difficult. So, we use the log-rank test.
The idea here is to test the null hypothesis, H0, that the expected value of the random variable X, E(X) = 0, and to build a test statistic of the following form,
X is the sum of the number of people who died at each time.
R does the job for you; use the library, survival.
library(ISLR2)
attach(BrainCancer)
as_tibble(BrainCancer)
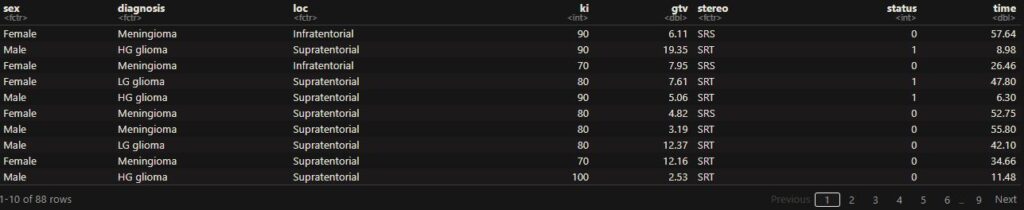
library(survival)
survdiff(Surv(time, status) ~ sex)
Call:
survdiff(formula = Surv(time, status) ~ sex)
N Observed Expected (O-E)^2/E (O-E)^2/V
sex=Female 45 15 18.5 0.676 1.44
sex=Male 43 20 16.5 0.761 1.44
Chisq= 1.4 on 1 degrees of freedom, p= 0.2
p = 0.2; we cannot reject the null hypothesis of no difference in survival curves between females and males.
Reference
An introduction to Statistical Learning: James, Witten, Hastie, Tibshirani, Taylor