One of the simpler explanations for Bayesian inference is given by John K. Kruschke in his book, Doing Bayesian Data Analysis. As per the author, Bayesian inference is the reallocation of credibility across possibilities. Let me explain what he meant by that.
Suppose there are four different, mutually exclusive causes for an event. And we don’t know what exactly caused the event to happen. In such cases, we may give equal credibility, 0.25, to each. This forms the prior credibility of the events. Imagine, after some investigations, one of the possibilities is ruled out. The new credibilities are now restricted to the three remainings, with the weightage automatically updated to 0.33. We call the new set posterior.
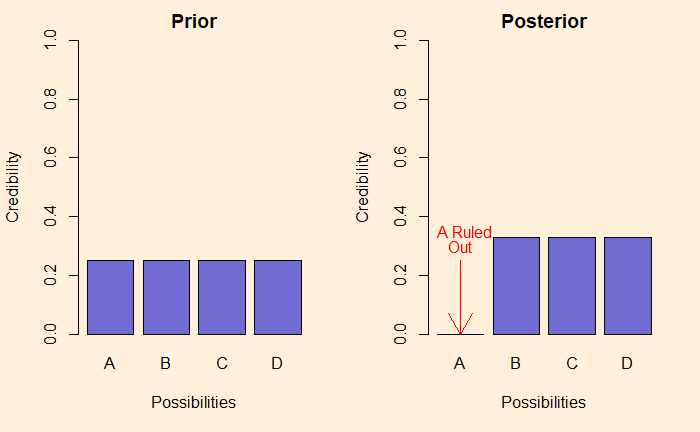
If you continue the investigation and eliminate one more, the situation becomes as shown below.

Notice the previous posterior is the new prior.
Reference
Doing Bayesian Data Analysis by John K. Kruschke